SDFD: Building a Versatile Synthetic Face Image Dataset with Diverse Attributes
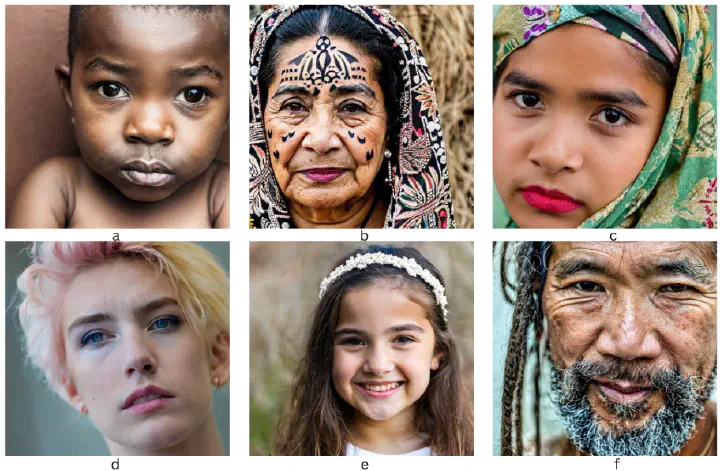
Artificial Intelligence (AI) systems today are incredibly advanced, leveraging vast amounts of data to perform complex tasks with high accuracy. These systems require huge datasets to train effectively. The quality and quantity of data significantly impact their performance, enabling them to recognize patterns, make predictions, and improve decision-making processes. As AI continues to evolve, the demand for large, diverse, and high-quality datasets increases especially, for image-based systems dealing with demographic attribute prediction that often face considerable bias and discrimination issues. Many current face image datasets primarily focus on representativeness in terms of demographic factors such as age, gender, and skin tone, overlooking other crucial facial attributes like hairstyle and accessories. This narrow focus limits the diversity of the data and consequently the robustness of AI systems trained on them.
In our recent work “SDFD: Building a Versatile Synthetic Face Image Dataset with Diverse Attributes” that we presented in the 1st International Workshop on Responsible Face Image Processing, we tried to address the former limitations of existing datasets, by proposing a methodology for generating synthetic face image datasets that capture a broader spectrum of facial diversity. Specifically, our approach integrates a state-of-the-art text-to-image generation model with a systematic prompt formulation strategy, encompassing not only demographics and biometrics but also non-permanent traits like make-up, hairstyle, and accessories. These lead to a comprehensive dataset of high-quality realistic images, which can be used as an evaluation set in face analysis systems.
The first step in our process was to compile a list of terms that will represent the attributes that one would like to be depicted in the target face images. These terms should be carefully picked and then filtered to eliminate specific words or phrases (see Table 1). After completing the basic attribute list, combinations of them are created to generate the text prompt. Prompts are used as input text to the generative model, describing the image that should be constructed. The more exact the description, the closer the resulting image will be to what was envisioned. Besides, negative terms are also important in the prompt process since they specify what should not be included in the generated image.
Figure 1 shows some example face image datasets created by our methodology along with their input prompts.
We opted for Stable Diffusion v2.1 [1] and generated 1000 face images, which constitute the first version of our Stabe Diffusion Face-image Dataset (SDFD).
We compared SDFD with the existing FairFace [2] and LFW [3] datasets, which are established face images that comprise real images and are used in several fairness-aware research works. Our comparative study demonstrated that SDFD was an equally or even more challenging dataset compared to the existing ones, while also being considerably smaller in size.
In the future, we intend to extend the number of images in our dataset to include less well-represented facial attributes such as scars, braces, and even disabilities - that we were unable to include in the current dataset version due to limitations in the text-to-image model. Besides, we intend to explore different prompting strategies and generative models. Finally, we would also like to extend SDFD with multiple images for the same identity to make it appropriate for other tasks such as face verification.
SDFD is publicly available on Github and the corresponding paper on arXiv.
References
[1] Rombach, R., Blattmann, A., Lorenz, D., Esser, P., & Ommer, B. (2022). High-resolution image synthesis with latent diffusion models. In Proceedings of the IEEE/CVF conference on computer vision and pattern recognition (pp. 10684-10695).
[2] Karkkainen, K., & Joo, J. (2021). Fairface: Face attribute dataset for balanced race, gender, and age for bias measurement and mitigation. In Proceedings of the IEEE/CVF winter conference on applications of computer vision (pp. 1548-1558).
[3] Huang, G. B., Mattar, M., Berg, T., & Learned-Miller, E. (2008, October). Labeled faces in the wild: A database for studying face recognition in unconstrained environments. In Workshop on faces in ‘Real-Life’ Images: detection, alignment, and recognition.
The content of this post is licensed under a Creative Commons Attribution-NonCommercial-ShareAlike 4.0 International License (CC BY-NC-SA 4.0).