Overcoming Unimodal Bias in Multimodal Misinformation Detection
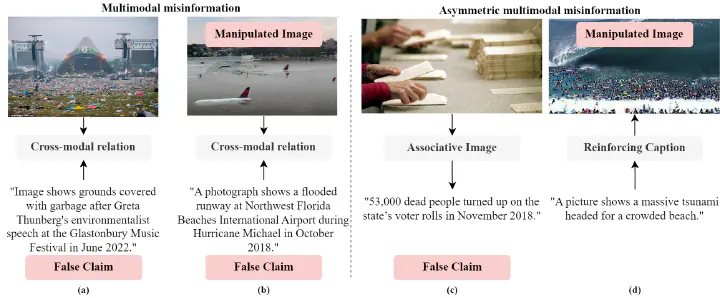
In this post, we explain the basics behind our paper “VERITE: a robust benchmark for multimodal misinformation detection accounting for unimodal bias”, by Stefanos-Iordanis Papadopoulos, Christos Koutlis, Symeon Papadopoulos and Panagiotis C. Petrantonakis, which has been published in the International Journal of Multimedia Information Retrieval (IJMIR).
Given the rampant spread of misinformation, the role of fact-checkers becomes increasingly important and their task more challenging given the sheer volume of content generated and shared daily across social media platforms. Studies reveal that multimedia content, with its visual appeal, tends to capture attention more effectively and enjoys broader dissemination compared to plain text (Li & Xie, 2019) and the inclusion of images can notably amplify the persuasiveness of false statements (Newman et al., 2012). It is for that reason that Multimodal Misinformation (MM) is very concerning. MM involves false or misleading information disseminated through diverse “modalities” of communication, including text, images, audio, and video. Scenarios often unfold where an image is removed from its authentic context, or its accompanying caption distorts critical elements, such as the event, date, location, or the identity of the depicted person. For instance, in the above figure (a) we observe an image where the grounds of a musical festival are covered in garbage, accompanied by the claim that it was taken in June 2022 “after Greta Thunberg’s environmentalist speech”. However, the image was removed from its authentic context, since it was actually taken in 2015, not 2022.
In response, researchers have been developing a range of AI-based methods for detecting MM in recent years. Typically, AI-based approaches for MM detection require two things: 1) a sufficiently large training dataset, and 2) deep learning model(s) capable of analyzing and recognizing patterns across both visual and textual modalities. Yet, the process of collecting and annotating a large dataset is cumbersome, time-consuming, and resource-intensive. To circumvent this problem, researchers have been experimenting with the creation of algorithmically created or “synthetic” misinformation. This synthetic data aims to mimic, to a certain extent, the characteristics of real-world misinformation, facilitating the training of deep learning models. Subsequently, to assess the models’ performance and their ability to generalize to authentic instances of misinformation, researchers evaluate them using annotated datasets featuring real-world examples of MM (Papadopoulos et al., 2023).
In our recent research paper, we uncovered the existence of unimodal bias within widely used evaluation benchmarks for MM detection, specifically VMU Twitter (MediaEval2016) and COSMOS. The term “unimodal bias” denotes the phenomenon where a model relying solely on one modality (text-only or image-only) appears to outperform its multimodal counterpart in what is supposedly a multimodal task. This observation raised a few critical questions: what are the underlying reasons for unimodal bias? Can we reliably assess progress in the field of MM detection with these datasets? If not, how can we create a new evaluation benchmark that effectively addresses the challenge of unimodal bias?
First and foremost, we posit that a key contributor to unimodal bias is what we term “asymmetric multimodal misinformation” (Asymmetric-MM). We introduce the term Asymmetric-MM to emphasize cases where a single “dominant” modality plays a pivotal role in disseminating misinformation, while other modalities exert minimal or no influence. Illustratively, in the above figure (c) a claim about “deceased people turning up to vote” is accompanied by an image that is thematically related to the claim, which primarily serves a cosmetic purpose. By concentrating on the dominant modality, a robust text-only detector would effectively identify misinformation, making the contribution of the other modality negligible in the detection process.
Furthermore, we introduce the concept of “modality balancing” as a proactive measure to counteract unimodal bias. In this framework, all images and captions are included twice during the evaluation process – once in their truthful pair and once in their misleading pair. The underlying rationale is to necessitate that a model consistently considers both modalities and their interrelation when discerning between truthfulness and misinformation. By adopting this approach, we aim to prevent text-only and image-only detectors from achieving seemingly high accuracy solely by focusing on one modality.
By leveraging modality balancing, excluding Asymmetric-MM and establishing rigorous criteria, we curated data from reputable fact-checking sources, namely snopes.com and reuters.com/fact-check, and created the VERITE benchmark, which stands for “VERification of Image-TExt pairs”. The above figure illustrates the data collection process for creating VERITE. Starting from articles centered around “Miscaptioned Images” and adhering to a set of inclusion criteria, we identified the authentic claim related to the image (depicted by continuous blue lines) and juxtaposed it with the false “miscaptioned” caption (MC) (illustrated by intermittent red lines). Subsequently, we formulated a query associated with the truthful claim to retrieve a related but out-of-context (OOC) image (depicted by dotted yellow lines). Thus, VERITE consists of three categories: Truthful, Out-of-context, and Miscaptioned image-caption pairs and can be used both for multiclass and binary classification tasks. We collected 260 articles from Snopes and 78 from Reuters that met our criteria resulting in 338 Truthful, 338 Miscaptioned and 324 Out-of-Context pairs.
We undertook a comprehensive comparative analysis based on a Transformer-based architecture trained on various established training datasets as well as a novel method of our own for algorithmically created training data. Our findings demonstrate that VERITE successfully mitigates unimodal bias, making it a robust evaluation benchmark and more reliable as a means of assessing progress in the field of MM detection. Our proposed method, which is a state-of-the-art deep learning method, reached up to 72.7% accuracy for “out-of-context” pairs, 63.9% for “miscaptioned images” and 52.1% overall accuracy for the multiclass classification task. This leaves ample room for further experimentation and improvement for the task of MM detection and we have provided a series of different routes that future research could explore. VERITE is publicly available on zenodo and github.
References
[1] Li Y, Xie Y (2020) Is a picture worth a thousand words? an empirical study of image content and social media engagement. J Mark Res 57(1):1–19.
[2] Newman EJ, Garry M, Bernstein DM et al (2012) Nonprobative photographs (or words) inflate truthiness. Psychon Bull Rev 19:969–974.
[3] Papadopoulos, S. I., Koutlis, C., Papadopoulos, S., & Petrantonakis, P. (2023, June). Synthetic Misinformers: Generating and Combating Multimodal Misinformation. In Proceedings of the 2nd ACM International Workshop on Multimedia AI against Disinformation (pp. 36-44).
The content of this post is licensed under a Creative Commons Attribution-NonCommercial-ShareAlike 4.0 International License (CC BY-NC-SA 4.0).