Mitigating Viewer Impact From Disturbing Imagery Using AI Filters A User-Study
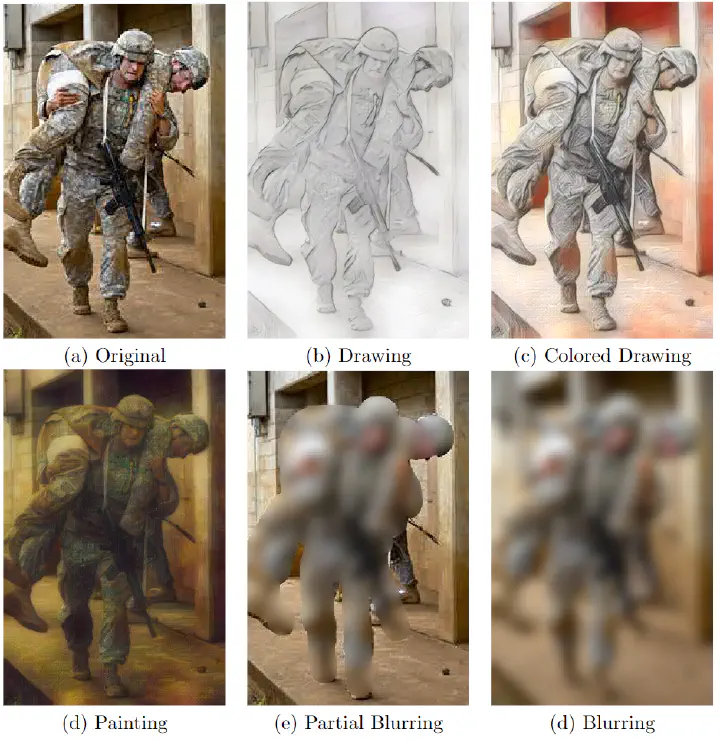
In today’s digital age, professionals such as journalists and human rights investigators often encounter distressing and graphic imagery as part of their work. The emotional toll of viewing such content can be profound, leading to secondary trauma and affecting mental well-being. Traditional methods like blurring have been used to shield viewers from explicit details, but they often compromise image interpretability.
Exploring The Capabilities of AI filters
Our recent paper “Mitigating Viewer Impact From Disturbing Imagery Using AI Filters A User-Study” explores the effectiveness of Artificial Intelligence (AI) filters on mitigating the emotional impact of disturbing imagery while preserving crucial image information.
AI has revolutionized various fields, including image processing. Style transfer techniques, which alter image appearance based on artistic styles, offer a promising alternative to traditional blurring. Unlike blurring, which can obscure details crucial for professional analysis, AI filters can maintain image integrity while reducing negative emotional responses significantly. Our study, involving 107 participants primarily from journalism and human rights fields, assessed the performance of five filter styles: Blurring, Partial Blurring, Drawing, Colored Drawing, and Painting.
Study Findings: The Power of AI Filters
Participants reported a remarkable -30.38% reduction in negative emotional impact when viewing images filtered with the AI-based Drawing style. Moreover, this style retained an impressive 97.19% of image interpretability, far surpassing the 6.54% retained by traditional Blurring techniques. Furthermore, professionals highlighted the potential of AI filters as a preparatory step before viewing original images. This approach could mitigate immediate emotional distress while allowing for detailed inspection when necessary. Participants also suggested enhancements and identified workflow stages where AI filters could be seamlessly integrated.
To delve deeper into our findings and explore the future of AI in content moderation, read our full study here.
The content of this post is licensed under a Creative Commons Attribution-NonCommercial-ShareAlike 4.0 International License (CC BY-NC-SA 4.0).