FRCSyn-onGoing:Benchmarking and comprehensive evaluation of real and synthetic data to improve face recognition systems
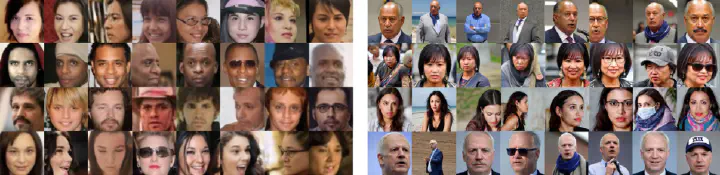
This post provides an overview of the paper “FRCSyn-onGoing: Benchmarking and comprehensive evaluation of real and synthetic data to improve face recognition systems” , which has been published in the Information Fusioninformation-fusion journal. This has been a collaborative effort led by the organizers of the FRCSyn-onGoing challenge and several of the participating teams, such as ours.
The utilization of synthetic data in face recognition systems offers several advantages over real-world datasets. Synthetic datasets provide a solution to privacy concerns associated with collecting real data that potentially include personal information without obtaining the consent by the affected individuals, a case that is quite common in image datasets sourced from the Internet. They also enable the generation of large datasets, which is especially important after the discontinuation of established databases due to privacy issues and regulations like the General Data Protection Regulation (GDPR) [27]. Synthetic data can be tailored to specific characteristics, such as demographic groups and age, without the need for additional human efforts, unlike real-world databases that may lack diversity in representation or suffer from a variety of sampling biases.
In particular, the paper focuses on advancing face recognition technology by evaluating the effectiveness of using synthetic data alongside real data. This initiative involves a collaborative effort from researchers worldwide to address the limitations and challenges faced by current face recognition systems. By combining real and synthetic data, the paper aims to enhance the performance and accuracy of face recognition technology. The article summarizes the findings from the Face Recognition Challenge in the Era of Synthetic Data (FRCSyn) held at WACV 2024.
One of the key aspects of the paper is the comprehensive evaluation of Face Recognition (FR) systems across different demographic groups and databases. Researchers have identified notable findings, such as lower performance on demographic groups representing the Asian population compared to others. However, training face recognition systems with synthetic data generated by frameworks like DCFace [1] and GANDiffFace [2] (see Figure 1) has led to promising results, particularly in addressing demographic diversity within test populations.
Teams participating in the challenge, among which the MeVer team, proposed new approaches to reduce demographic bias and improve the overall performance of FR systems. By using ResNet backbones, ArcFace, and AdaFace loss functions, with several integrating multiple networks and advanced data augmentation techniques, researchers aimed to create less biased features and enhance the learning process for FR systems. Standardized benchmarks and metrics to evaluate FR performance in realistic scenarios, are also presented. By considering factors such as pose variations, aging, occlusions, and diverse demographic groups, researchers provide a more comprehensive assessment of the capabilities of these systems.
Through the FRCSyn-onGoing challenge, researchers are paving the way for the future of FR technology by exploring the potential of synthetic data and domain generalization techniques. The collaboration between industry and academia in this initiative highlights the importance of addressing demographic bias and improving the fairness and accuracy of face recognition systems. By continuing to explore the benefits of synthetic data and innovative approaches, the paper aims to drive advancements in FR technology and contribute to rendering it more trustworthy and reliable.
References
[1] M. Kim, F. Liu, A. Jain, X. Liu, DCFace: Synthetic face generation with dual condition diffusion model, in: Proceedings of the IEEE/CVF Conference on Computer Vision and Pattern Recognition, 2023.
[2] P. Melzi, C. Rathgeb, R. Tolosana, R. Vera-Rodriguez, D. Lawatsch, F. Domin, M. Schaubert, GANDiffFace: Controllable generation of synthetic datasets for face recognition with realistic variations, in: Proceedings of the IEEE/CVF International Conference on Computer Vision Workshops, 2023.
[3] P. Voigt, A. Von dem Bussche, The EU general data protection regulation (GDPR), in: A Practical Guide, first ed., Vol. 10, (3152676) Springer International Publishing, Cham, 2017, pp. 10–5555.
The content of this post is licensed under a Creative Commons Attribution-NonCommercial-ShareAlike 4.0 International License (CC BY-NC-SA 4.0).