Unveiling AI Bias in Facial Analysis: Meet FaceX
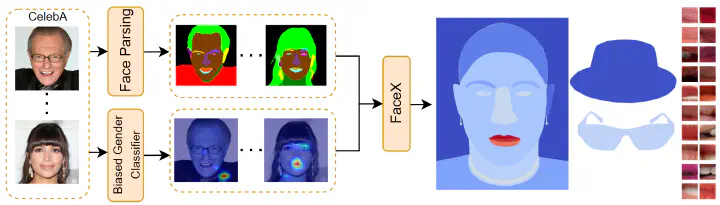
In today’s world, AI systems are integral to many applications, from unlocking smartphones to recognizing emotions. While these advancements bring numerous benefits, they also raise ethical and societal concerns, particularly regarding AI bias. Facial analysis technologies, in particular, have been scrutinized for their potential biases, which can disproportionately affect certain groups of people. This is where our new tool, FaceX, comes into play.
What is FaceX?
FaceX is a novel method designed to uncover biases in facial attribute classifiers. While existing instance-level XAI methods require labor-intensive manual inspections to draw general conclusions about model behavior, FaceX addresses this limitation by aggregating model activations at the region level, making it easier to visualize and understand biases. In particular, it summarizes model behavior across 19 predefined facial regions, such as hair, ears, and skin, providing a more comprehensive understanding of where and why a model focuses on specific facial areas.
How Does FaceX Work?
FaceX computes a region-level aggregation of model instance-level attributions, summarizing the model’s output with respect to each region of interest. Then, spatial explanations, offered through a heatmap visualization over an abstract face prototype, provide in-depth understanding of the weight of each facial region (or accessory) on the model decision. Additionally, FaceX visualizes the high-impact image patches for each region, revealing not only where the model focuses but also helping the human analyst understand why certain features are influential. This dual approach of spatial explanation (understanding where the model focuses) and appearance-oriented insights (understanding the impact of specific image patches) sets FaceX apart as a powerful tool for identifying biases in facial analysis systems and acts as a comprehensive lens, allowing practitioners, researchers, and developers to scrutinize the entire spectrum of model behavior.
Want to dive deeper? Check out the paper and run FaceX to explain the behaviour of your models.
The content of this post is licensed under a Creative Commons Attribution-NonCommercial-ShareAlike 4.0 International License (CC BY-NC-SA 4.0).