Deepfakes: An Emerging Internet Threat and their Detection
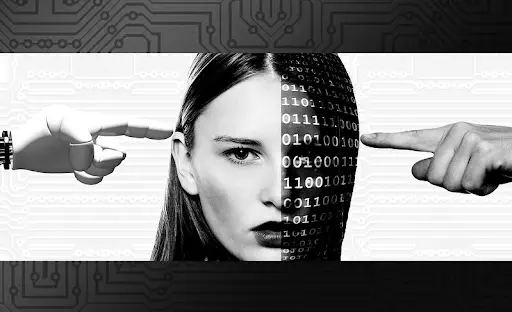
Towards the end of 2020, I had the opportunity to give a talk on Deepfakes in the 60th AI4EU WebCafe. With deepfakes being already with us for more than two years now, we have already witnessed several real-world cases of deepfakes with both positive and negative impact, while their use has recently been commoditized by popular apps such as ZAO and Reface. At the same time, it seems that there is an exponentially growing interest in the area from the academic community as indicated by the quickly growing number of papers related to the topic.
Even though convincing deepfake generation is still hard and resource-intensive (both in terms of computing power and manual video editing skills), there has been immense progress in the area and numerous approaches and techniques that have led to remarkable improvements in the quality and realism of the resulting deepfakes, as well as on the requirements in terms of number of training samples and compute power. The following image shared by Ian Goodfellow, the father of Generative Adversarial Networks (GANs) vividly illustrates the progress achieved during the last years.
TWEET
What is more, there is now a variety of open-source tools and repositories, with FaceSwap and DeepFaceLab being the most popular ones, that have considerably lowered the barrier to entry even to non-experts (but still a bit tech-savvy).
Following the progress of deepfake generation approaches, there has been commensurate progress in the inverse problem of deepfake detection. There have been numerous types of approaches tackling the problem. A first class of such approaches try to spot deepfake artifacts, much like a careful human inspector would, for instance, fuzzy and blurry areas around the lips, earlobes and hair, lack of symmetries, e.g. different colors between the left and right eye, fuzzy background, etc. There are also approaches that attempt to extract physiological signals from a video in question, e.g. eye blinking or human pulse, and detect whether there are inconsistencies or unrealistic patterns in their evolution. Other approaches adopt a more general supervised learning paradigm, trying to learn distinctive deepfake patterns by looking into sets of both deepfake and authentic images. Last but not least, as in the case of media forensics approaches, there are deepfake detection methods that try to uncover deepfake artifacts in the frequency domain.
Even though the area is quite young, there are already a few very good surveys on the topic:
- Media Forensics and DeepFakes: an overview by Verdoliva
- DeepFakes and Beyond: A Survey of Face Manipulation and Fake Detection by Tolosana et al.
- The Creation and Detection of Deepfakes: A Survey by Misky and Lee
Another great landmark for deepfake detection research has been the launch and completion of the DeepFake Detection Challenge. The challenge attracted more than 2100 participants and offered a very hard benchmarking setting, which revealed the main weakness of state-of-the-art deepfake detection methods, i.e. their limited ability to generalize to new types and forms of deepfake content. Yet, at the same time, the challenge pushed the performance boundary of solutions and raised awareness about new detection approaches to the community. Our MeVer team also participated in the challenge in the context of the WeVerify project and was quite successful, ranked among the top 5% of submitted solutions. We had already provided an overview of our solution in a previous blog post. Since then, we have also performed a number of improvements after releasing an alpha version of our service to our partners in the WeVerify project. You can get a glimpse on how our latest deepfake detection service looks in our latest blog post about verification tools, where we present all tools we developed so far in WeVerify.
Closing, my thoughts on the current state of deepfakes are the following:
- Deepfake generation still requires a certain degree of expertise and the resulting deepfakes are in most cases still possible to detect by a trained human observer, but this is likely to change in the near future (2-3 years), making possible the creation of highly realistic deepfakes with very little manual intervention and computational resources.
- There are a variety of deepfake detection approaches, each working well on different kinds of deepfakes. A common issue among all of them is the limited generalization ability to cases that were not well represented in the training set. However, there are certain approaches that claim to be general (e.g. those based on analysis of physiological signals and analysis on the frequency domain), at least given the current state of deepfake generation technology.
- Currently, the negative impact of deepfakes has mainly affected individuals, e.g. revenge porn, cyberbullying, while there have also been cases where there was broader socio-political impact as a result of deepfakes or even the suspicion of deepfakes (e.g. Gabon president video).
- We shouldn’t be fooled by the fact that there has been limited use of deepfakes in the context of disinformation campaigns to date. This is likely not due to the limitations of the technology but probably due to the fact that there are already established disinformation techniques that still work effectively and do not require any technical expertise. In the near future, however, this could change, so the risk of deepfakes for disinformation should not be underestimated. The video recording of the talk and the accompanying slides are available below.
VIDEO
SLIDES
The content of this post is licensed under a Creative Commons Attribution-NonCommercial-ShareAlike 4.0 International License (CC BY-NC-SA 4.0).