AI Fairness Definition Guide
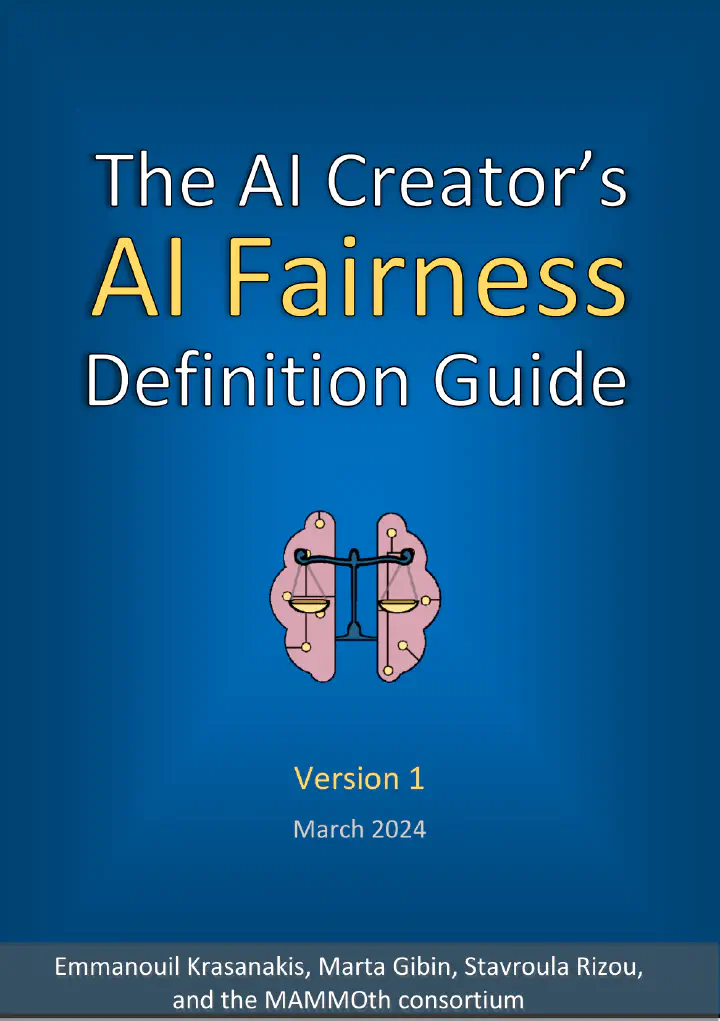
In the context of the Horizon Europe MAMMOth project, we developed the “AI Fairness Definition Guide” to help those creating AI (such as researchers, developers, and product owners) understand how to define fairness in the social context of their created systems by working with stakeholders and experts from other disciplines. The guide presents a workflow for gathering fairness concerns of affected stakeholders and using them to derive corresponding formalisms and practices under a combined computer, social, and legal science viewpoint.
The content of this post is licensed under a Creative Commons Attribution-NonCommercial-ShareAlike 4.0 International License (CC BY-NC-SA 4.0).